In an online labor marketplace employers post jobs, receive freelancer applications and make hiring decisions. These hiring decisions are based on the freelancer’s observed (e.g., education) and latent (e.g., ability) characteristics. Because of the heterogeneity that appears in the observed characteristics, and the existence of latent ones, identifying and hiring the best possible applicant is a very challenging task. In this work we study and model the employer’s hiring behavior. We assume that employers are utility maximizers and make rational decisions by hiring the best possible applicant at hand. Based on this premise, we propose a series of probabilistic models that estimate the hiring probability of each applicant. We train and test our models on more than 600,000 job applications obtained by oDesk.com, and we show evidence that the proposed models outperform currently in-use baselines. To get further insights, we conduct an econometric analysis and observe that the attributes that are strongly correlated with the hiring probability are whether or not the freelancer and the employer have previously worked together, the available information on the freelancer’s profile, the countries of the employer and the freelancer and the skillset of the freelancer. Finally, we find that the faster a freelancer applies to an opening, the higher is the probability to get the job.
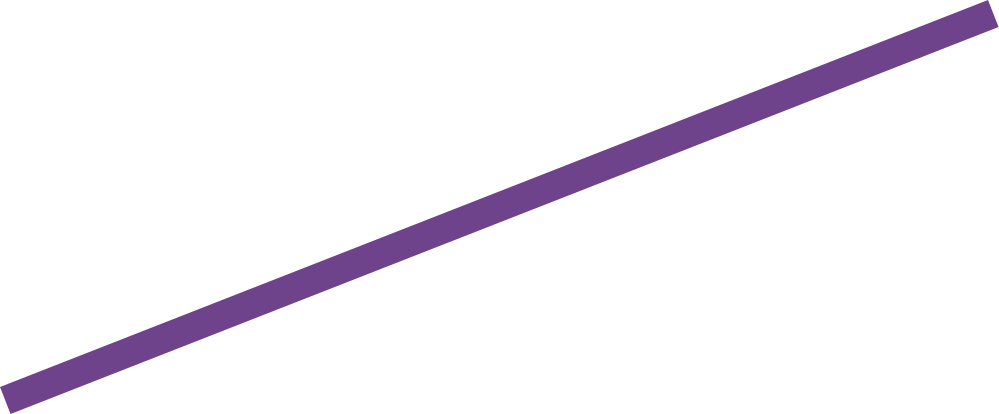
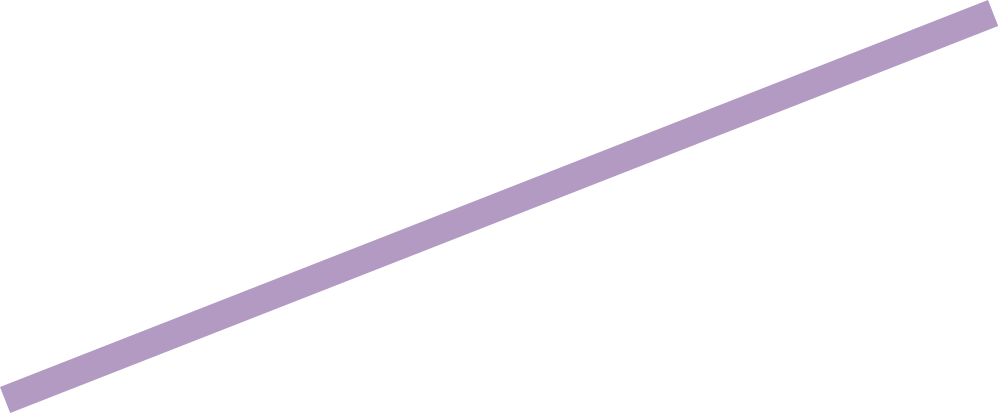


Hiring Behavior Models for Online Labor Markets
- Panagiotis Ipeirotis
- Marios Kokkodis
- Panagiotis Papadimitriou
- Venue: Eighth ACM International Conference on Web Search and Data Mining (WSDM 2015)
- Feb 2015
- Status: Refereed
- Type: Conference
- Acceptance rates: 40/238 = 16.8% accepted