In the last decade, prediction markets became popular forecasting tools in areas ranging from election results to movie revenues and Oscar nominations. One of the features that make prediction markets particularly attractive for decision support applications is that they can be used to answer what-if questions and estimate probabilities of complex events. Traditional approach to answering such questions involves running a combinatorial prediction market, what is not always possible. In this paper, we present an alternative, statistical approach to pricing complex claims, which is based on analyzing co-movements of prediction market prices for basis events. Experimental evaluation of our technique on a collection of 51 InTrade contracts representing the Democratic Party Nominee winning Electoral College Votes of a particular state shows that the approach outperforms traditional forecasting methods such as price and return regressions and can be used to extract meaningful business intelligence from raw price data.
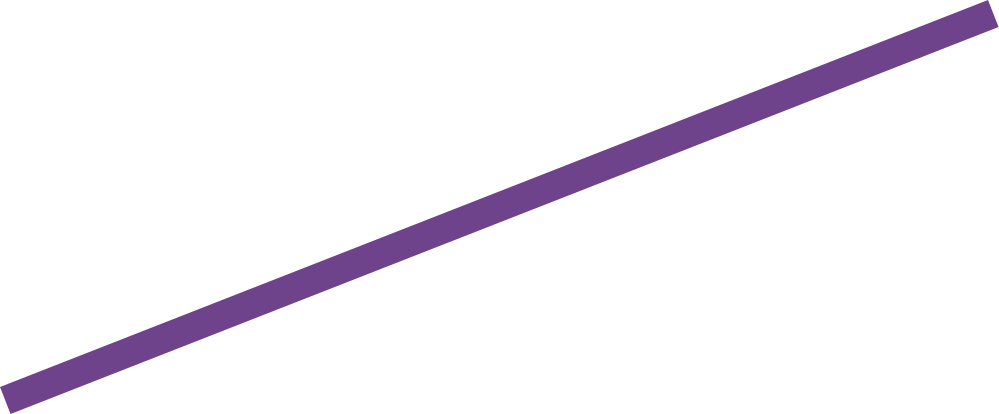
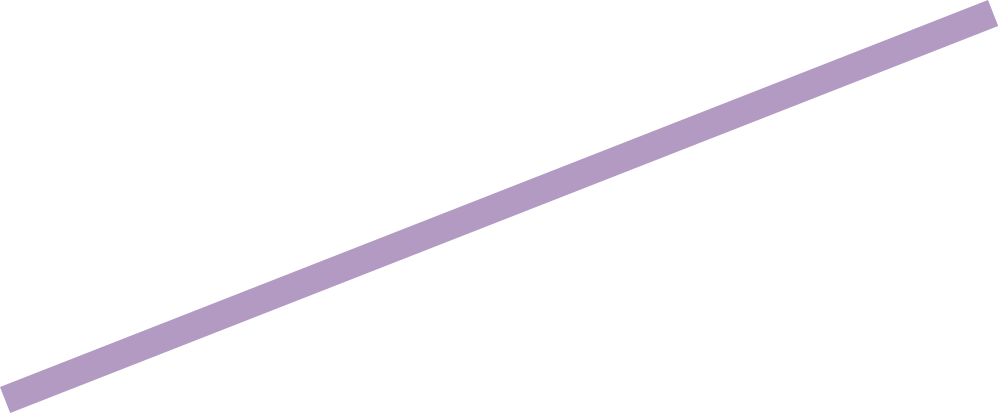


Modeling Dependency in Prediction Markets
- Nikolay Archak
- Panagiotis Ipeirotis
- Venue: NYU Center for Digital Economy Research Working Paper No. CEDER-10-05
- Dec 2010
- Status: Unrefereed
- Type: Working paper