Increasingly, user-generated product reviews, images and tags serve as a valuable source of information for customers making product choices online. An extant stream of work has looked at the economic impact of reviews. Typically, the impact of product reviews has been incorporated by numeric variables representing the valence and volume of reviews. In this paper, we posit that the information embedded in product reviews cannot be fully captured by a single scalar value. Rather, we argue that product reviews are multifaceted and hence, the textual content of product reviews is an important determinant of consumers’ choices, over and above the valence and volume of reviews. Based on a unique dataset of hotel reservations available to us from Travelocity, we estimate demand for hotels using a two-step random coefficient based structural model. We use text mining techniques that allow us to incorporate textual information from user review in demand estimation models by inferring the sentiments embedded in them and supplement them with image classification techniques. The dataset contains complete information on transactions conducted over a 3 month period from Nov – Jan 2009 for hotels in the US. We have data on user- generated content from three sources: (i) user-generated hotel reviews from two well known travel search engines, Travelocity and Tripadvisor, (ii) tags generated by users identifying different locational attributes of hotels from Geonames.org, and (iii) user contributed opinions on the most important hotel characteristics from Amazon Mechanical Turk. Moreover, since some location-based characteristics, such as proximity to the beach, are not directly measurable based on UGC, we use image classification techniques to infer such features from the satellite images of the area. These different data sources are then merged to create one comprehensive dataset that enables us to estimate the weight that consumers place on different hotel characteristics. We then propose to design a new hotel ranking and recommendation system based on the empirical estimates of consumer surplus from hotel transactions. By improving the recommendation strategy of travel search engines, it can raise the conversion rate for a particular hotel, hence increasing the return-on-investment for travel search engines.
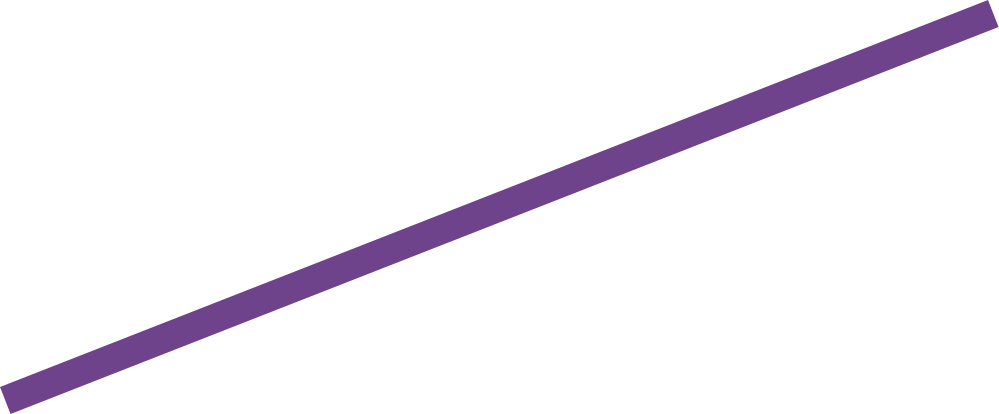
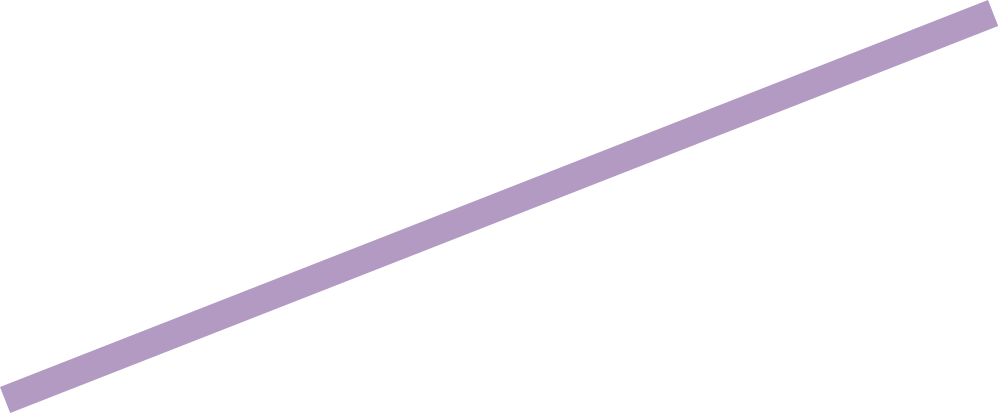


The Economic Impact of User-Generated Content on the Internet: Combining Text Mining with Demand Estimation in the Hotel Industry
- Anindya Ghose
- Panagiotis Ipeirotis
- Beibei Li
- Venue: Proceedings of the 20th Workshop on Information Systems and Economics (WISE), 2009
- Sep 2009
- Status: Refereed
- Type: Workshop